Predictive Analytics: An All-In-One Guide for a Data-Driven Future

In today's data-driven and analytical landscape, predictive analytics is revolutionizing decision-making processes across a large variety of competitive industries.
According to a 2023 survey by NewVantage Partners, 59.5% of organizations are utilizing predictive data analytics to inform decisions and drive business innovation forward.
Predictive analytics involves advanced algorithms and machine learning techniques to analyze historical data, understand patterns, and predict future trends. In this blog, you'll explore the concept of predictive analytics and its key components and shed some light on the future of this revolutionary technology.
Understanding Predictive Analytics
Predictive analytics utilizes enhanced data analysis techniques such as statistical modeling and machine learning algorithms to detect minute patterns and predict future outcomes. The process allows for the identification of relationships and correlations that may be beyond our own observations.
The automated nature of predictive analytics also makes it adaptable to different fields and datasets, utilizing a variety of techniques, such as decision trees and neural networks, to adjust for different data features and analysis objectives. According to a 2023 survey by NewVantage Partners, 91.9% of organizations were able to achieve measurable business value from data and analytics investments in 2023.
Predictive analytics can be used in fields like finance, marketing, healthcare, and beyond, giving organizations a powerful tool for the complex calculations required for tedious tasks such as resource allocation and risk mitigation.
The Key Components of Predictive Analytics
Predictive Analytics involves a series of complex mechanisms that play a vital role in converting raw data into actionable insights.
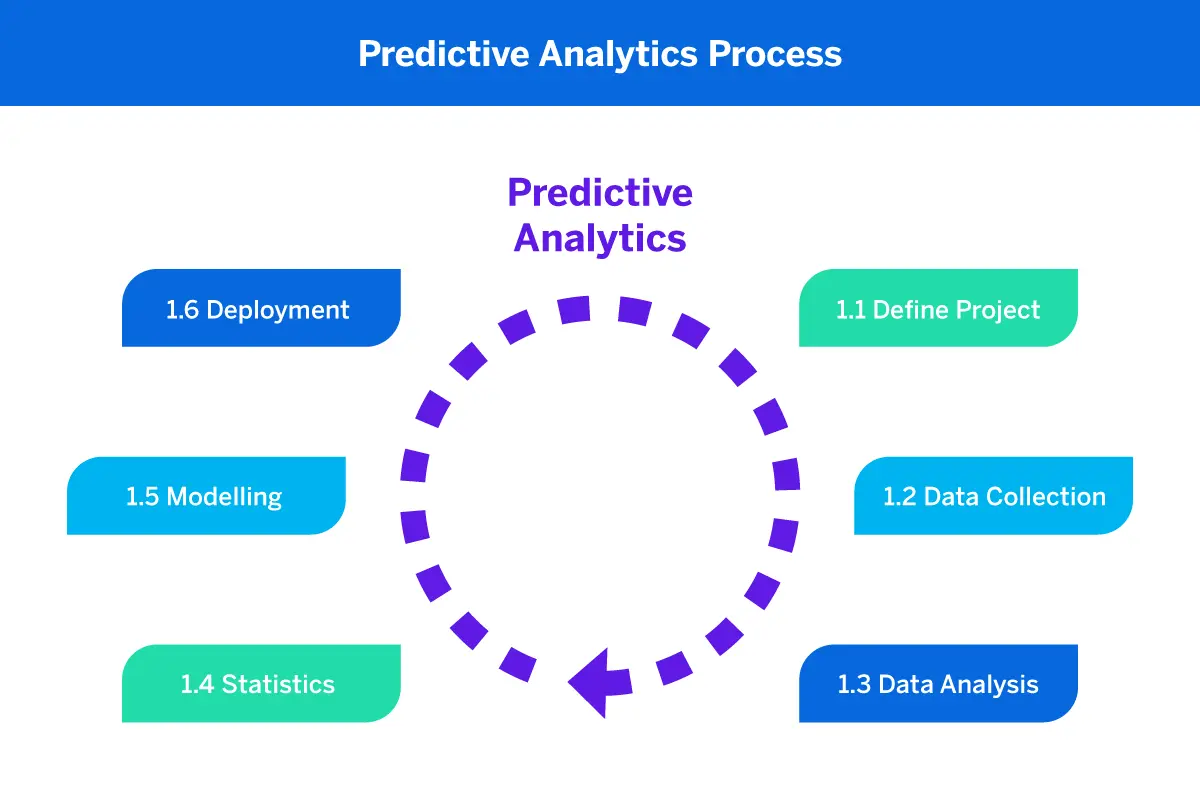
The ever-increasing adoption of the process makes it essential for us to understand the different components at play throughout. Let's take a look at the following:
1. Data Collection
The process of predictive analysis begins with the identification and sourcing of data from relevant channels. The quality of this data directly influences the predictive algorithm's accuracy, which is why comparing and evaluating datasets allows for a more comprehensive analysis of hidden patterns or relationships.
2. Data Preprocessing
After the collection of raw data, it goes through a preprocessing stage that involves cleaning and transforming the data into a structured format that can be further scrutinized. The resolution of any missing values and dealing with anomalies is crucial for ensuring that the data is suitable for a particular predictive model.
3. Feature Selection
Feature selection involves the selection of variables that contribute significantly to the model's predictive power while simultaneously getting rid of irrelevant data features. This optimization improves the model's performance and reduces the risk of overfitting.
4. Model Building
Model building is the stage where the appropriate predictive algorithm is selected based on the particulars of the problem that requires solving, the dataset and its features, and the objectives of the analysis. Techniques such as linear regression and complex methods for more intricate patterns help develop the predictive model's efficacy over time.
5. Training the Model
After the model is built, it is trained using historical data. This involves training the model with historical patterns in order to allow it to learn any underlying patterns and correlations within the data. The training phase allows for the refinement of the model so that it is able to understand the subtle nuances of datasets and can be generalized for use on new data.
6. Validation and Testing
The trained model undergoes testing and further refinements before it can be subjected to functioning in real-world scenarios. The testing and refinement process evaluates performance on separate datasets to ensure the model's reliability and its ability to make accurate predictions.
7. Prediction and Deployment
After a successful testing and refinement process, the model becomes ready to be used in a real-world environment to make real-time predictions on new data, integrating its powerful predictive capabilities into decision-making processes.
8. Continuous Improvement
Regular refinements of the model based on new data, changing trends, and evolving business strategies help the predictive model remain robust and adaptable over time. This iterative approach allows organizations to stay ahead in a dynamic and competitive environment.
Challenges in Predictive Analytics
Predictive analytics faces several challenges that need to be successfully circumvented for its successful implementation. Let's take a look at these challenges.

1. Data Quality and Quantity
The efficacy of a predictive model is foundationally dependent on the quality and quantity of the data it is developed upon. Insufficient or inaccurate data can negatively impact the model's ability to make reliable predictions.
2. Overfitting and Underfitting
Overfitting, where a model becomes too adjusted to its training data, can cause poor functioning when exposed to new data. On the other hand, Underfitting, wherein a model tends to oversimplify patterns, can cause a model to miss complexities present in the dataset.
3. Model Interpretability
Understanding how a model reaches a certain prediction is essential in sectors with regulatory or ethical requirements. Models that are accurate and also interpretable can involve compromises between complexity and transparency.
4. Changing Data Distributions
A predictive model trained on historical data might face challenges when applied to new datasets. Monitoring data changes and utilizing adaptive strategies helps maintain the model's relevance and accuracy over time.
5. Ethical and Bias Concerns
Predictive models can lead to incorrect outcomes due to any biases present in historical data. Ensuring fairness in model predictions requires continuous monitoring and addressing biases to minimize unintended outcomes.
6. Feature Selection and Engineering
Inappropriate feature selection can cause models to miss out on patterns, whereas features that are too complex can lead to overfitting. Strategic feature engineering is necessary for creating robust predictive models.
7. Optimal Model Selection
Choosing the optimal algorithm depends on the specific problem that requires resolution, the specifics of the dataset, and all analysis objectives. Informed decision-making helps organizations avoid subpar model performance.
8. Limited Domain Expertise
A lack of expertise can negatively affect the development of effective predictive models. Productive collaboration between data scientists and knowledge workers is essential for bridging the information gap.
9. Deployment and Integration
Upon applying models in real-world environments, challenges can arise when fitting predictive models within operational processes. This necessitates collaboration between data science and IT teams to ensure proper functioning.
10. Continuous Monitoring and Maintenance
Predictive models require constant monitoring and maintenance, and any changes in data or other factors can impact the model's performance. Mechanisms for continuous monitoring and refinement are important for the model's sustainability.
The 5 Best Practices for Predictive Analytics
To overcome any challenges, organizations utilizing Predictive Analytics should follow the following practices.

1. Define Clear Objectives
Laying out clear objectives and the specific outcomes desired from predictive analytics provides a proper blueprint for the process. A well-defined objective ensures alignment between analytics processes and organizational goals.
2. Quality Data Matters
The quality of the input data impacts the accuracy and reliability of predictions. Organizations should prioritize data quality validation to ensure that data is accurate and complete, which is foundational to the success of a predictive model.
3. Appropriate Model Selection
Each algorithm has its strengths and weaknesses, and selecting an inappropriate model can cause improper results. A well-developed understanding of the problems and overall process can help inform which algorithm might be most appropriate.
4. Continuous Model Monitoring
Continuous monitoring of model behavior is essential to ensure that the model maintains its accuracy and relevance over time. Establishing monitoring mechanisms allows organizations to take timely action.
5. Ethical Considerations
Predictive analytics can involve sensitive data, which means that organizations must take ethical considerations into account for the analytics process. Adhering to ethical standards helps develop trust and reduces the risk of unintended outcomes.
Applications of Predictive Analytics Across Industries
Predictive Analytics has diverse applications for driving innovations and elevating decision-making. Its impact can be seen in the following sectors:
1. Financial Forecasting
In the finance industry, predictive analytics are utilized to analyze historical data sets. These analytics serve as a valuable tool for predicting market trends, managing risks, and optimizing portfolios. Financial institutions can use this information to make well-informed decisions about investment strategies and risk reduction.
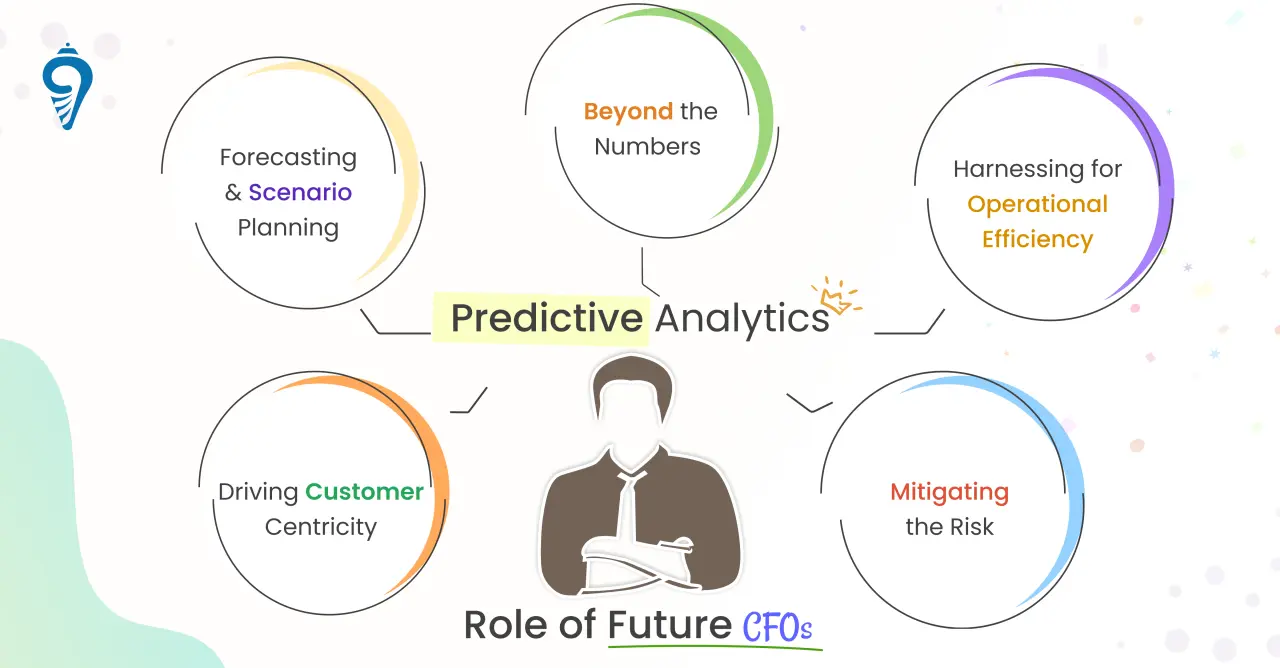
2. Marketing Campaigns
Marketers use predictive analytics to refine strategies and target their desired audiences. Analyzing customer behaviors and preferences allows for optimizing marketing campaigns and predicting future trends, which can lead to more effective customer acquisition and retention.
3. Healthcare
In healthcare, predictive analytics can analyze patient data to identify potential health risks, allowing healthcare providers to take action early and personalize treatment. Thus, predictive analysis for healthcare prediction has the potential to change traditional healthcare services.

Future Trends and Innovations
Advancements in technologies such as artificial intelligence and machine learning, will further enhance predictive capabilities, highlight the future of predictive analytics. The advent of real-time data processing will enable more dynamic predictions.
Ethical considerations are imperative for the future development of responsible AI, helping address biases and ensuring transparency. As predictive analytics increasingly becomes an integral part of decision-making processes, it will lead to a more contextually aware and data-driven future.
Conclusion
Navigating the complex components, challenges, and practices of predictive analytics can be daunting. However, its iterative nature drives revolutionary innovations, allowing businesses to confidently predict future trends and manage uncertainties in a rapidly changing world.
With predictive analytics, businesses can transform raw data into valuable insights, identifying patterns and trends that inform strategic decisions. Tools like Markov enhance this process by automating data analysis, reducing human error, and increasing productivity. By leveraging AI, organizations can stay ahead of the curve, anticipating market changes and making proactive decisions to navigate the future with confidence.
Experience the power of predictive analytics with Markov and unlock the full potential of your data to drive innovation and growth.
Book a demo today!
Let’s Talk About What MarkovML
Can Do for Your Business
Boost your Data to AI journey with MarkovML today!