How Marketing Teams Should Actually Adopt AI?
.png)
Isn't ChatGPT enough for all my marketing needs?
What's the difference between an LLM and an AI Agent?
As a marketing team, how do you use AI?
When this question is posed, the answer generally takes the form of: "Yes, we actively use ChatGPT," or "We use a combination of Perplexity, Gemini, or Claude, depending on the use case," and sometimes the answer is:
"We're starting to use dedicated solutions that have built-in AI for content creation or outbound personalization." Bravo!
The natural next question becomes, "How does the whole marketing team embrace AI for those specific use cases, and are they seeing value"?
The answer to this specific question is some combination of "It depends" or "We're actually just starting to figure that out".
As with any new technology, proficiency and use across the full team means you've completed some early experiments and seen positive results, and now you need to spend time to onboard, learn, and adapt from your existing processes and tools.
That skill-gap learning and time-to-value requires an iterative approach and a commitment to bring everyone along.
Focus on the Priority, Then Start to Embrace AI Solutions
It also comes down to prioritizing what's already on the plate or committed to. Most marketing teams have little bandwidth and are often trying to remove workloads in order to focus or "double down" on what's performing and delivering value.
And naturally, it must be safe and private, plus cost-effectively scale. This may seem like an obvious requirement, but it is important in this context as free LLM usage can be risky and scary for a corporate compliance and privacy team.
What's the Difference Between an LLM and an AI Agent?
Let's get started with definitions. Large Language Models (LLMs) are advanced artificial intelligence systems designed to process and generate sequential patterns like human language.
As we know, these models are trained on vast amounts of text data, allowing them to learn patterns, nuances, and complexities of any language.
These models perform a variety of language-based tasks, such as translation, summarization, and conversation generation.
The adoption of LLMs such as OpenAI's GPT-4.0/5.3 took off extremely fast, and now we use it for all kinds of actions in our daily lives, plus outside of work. It has also replaced Google search for many queries. Start with a good prompt, and off you go.
Marketers have fallen in love with LLMs' ease of use, instant results, low cost, and the ability to create content and answers extremely fast, using the right prompts.
Common uses include outlining a blog, getting answers to specific topics, as a thought starter, or structuring an outline for a long-form asset.
As models mature, we can start to do other transactional-type things, like create an image.
So, What's an AI Agent for?
In contrast to an LLM, an AI Agent is a system or software that uses AI to automate, enhance, or analyze specific tasks and processes.
Agents can perform a variety of functions such as customer data analysis, personalization of messages that are context aware or convert a video into a blog post and create social copy to promote across various channels.
Technically, AI agents are AI systems that can analyze a situation, decide, and take actions autonomously by designing an AI workflow and utilizing the arsenal of tools at their disposal.
For example, given a task, a "researcher" AI agent will break down the request into various sub-queries, identify what tools it can use to find answers to those sub-queries, assemble the response from all the tools, and verify that the response answers the original query or prompt. It then formats it in a specific way for the user to "consume".
Without an AI Agent, this workflow would take a human days, if not weeks, to accomplish, and by triggering an AI agent, the task can be done in minutes.
Why are LLMs Alone not Enough for Marketing Teams?
Of course, the human evaluator will ensure that data is correct and make any adjustments.
A concrete example may be the answer to the question:
"Why are my sales down this quarter?".
This query prompt is broken down into multiple sub-questions:
1. What is the timeline of the current and previous quarters?
2. What were total sales in the previous quarter?
3. What was the ACV of all sales?
4. What are the current sales numbers?
5. Which customers were sold to in the previous quarter?
6. What customers are in the pipeline in the current quarter?
7. How did we engage with customers and so on?
All this information is in different systems, and an agent will be trained to figure out which system to query to fetch the relevant information and how.
It will take care of breaking the query and calling these systems to get the information and more to answer the question, why my sales are down this quarter (based on available information).
AI agents help marketing professionals to efficiently perform such repetitive tasks that were previously manual and time-consuming.
Other examples for a marketer include:
- Account research: Target the right persona based on ICP criteria
- Competitive knowledge: understand how top competitors are marketing and selling,
- Competitive SEO: What keywords are your competitors using in their latest content and messages?
AI Agents will do the multi-steps rather than a transaction-based response from one prompt. Agents are your operators that perform all the sub-steps in order to speed up workloads or a set of end-to-end tasks.
Wait, Don't AI Agents also use LLMs?
Yes, in order to automate any task, having an LLM operate that task is exactly how the agent performs its work and output. Think of this as Data In (or in the context of some asset such as PDF, CSV, TXT) and then perform an operation, with a result output ready for download.
Some automation has multiple steps depending on what you need to accomplish.
As the user, you review the output as the "human in the loop" before any final publishing or external sharing.
That practice is not only commonplace but advisable for many reasons. More on that in a future blog post.
The key difference with a purpose- built AI Agent software system is you can select the best or optimal LLM to perform the operation.
You should not be forced to use that one LLM that you had previously used as a single tool. Inside a software solution, you select from various LLMs, which not only impact the result output but also, importantly, the overall cost.
Save, Clone, Configure, And Schedule for a Future Run!
How AI Agents differ, assuming they're operable from inside a dedicated system, is that they are highly configurable for future use or "runs".
Rather than operating independently, you now collaborate as one team and see exactly the AI Agents or workflows, already run or in process.
You also access the results and, what's more, schedule to run a workflow next week or at the cadence you prefer.
An AI Agent (or set of tasks), often referred to as automated workflows, retains the history of what happened.
Everyone has full visibility to look back. The result is stored, which is further queryable. Now, the whole team benefits. It speeds up operations and cycle times. Everyone wins. Everyone learns.
One person. One LLM. No Visibility and Re-use
By contrast, individuals on the team that have experimented with LLMs to speed up their workloads are cut-pasting the output results and then putting them into a Google doc with a link or via a shared asset.
This alone is cumbersome and takes time. It lacks flow steps with full access and visibility for the whole team.
What's more, the user also has to save prompts in another system and continuously update them, and those are specific only to the one LLM being used.
Also, how does the team member know which LLM is the best for the job for the given task.
There are constant changes and updates across most LLMs, and while they are getting better, it is a moving target, and therefore, you may not use the right LLM for the task at hand.
For example, some people find Claude better at Writing, OpenAI good for reasoning or Gemini good for Document Processing.
Staying on top of this is a lot to ask of each team member, who is busy working with a variety of other tools every day.
Before long, tool bloat becomes an issue, and it is hard to track and extract group learning. It's also risky from an overall cost perspective.
What's the Risk of Overdependence on LLMs?
LLMs are great—until they start thinking for you. Let’s talk about where to draw the line.
1. Loss of Human Touch
If you rely too heavily on an LLM to craft messages, it can lead to content that lacks the nuanced understanding and emotional resonance only humans can provide.
While LLMs generate content quickly and in large quantities, they can't fully capture the subtle emotional cues and cultural contexts that resonate with diverse audiences.
Sure, they can help with brand tone, but you are essentially letting machines talk to more machines, and at some point, the human reader will tune out and disengage.
2. Reduced Creativity and Innovation
Marketers who depend too much on LLMs may find their creative skills atrophying over time.
LLMs mimic existing data and info, which means they recycle familiar patterns and ideas.
This stifles innovation and prevents the emergence of fresh, original concepts that are vital for a brand to stand out in a competitive market.
Regurgitating content that already exists vs coming up with original thoughts and ideas is never a good idea for building a strong, respected brand reputation.
3. Potential for Miscommunication
LLMs are designed to generate responses based on patterns in data they've been trained on, which can lead to inaccuracies or inappropriate content if the model encounters a scenario it hasn't been specifically trained to handle.
Such errors can damage a brand's reputation if misleading or offensive content reaches the public.
Of course, we always want a human in the loop before publishing the final piece.
4. Dependence on Technology
As marketing practitioners, we know the craft as both an art and a science. It's also the practice of creating, connecting, and measuring.
We are continually in this back and forth. Ask the data and systems if the campaign is producing a return and driving revenue.
The human-in-the-loop is critical.
In fact, we already have many humans in the loop reviewing, analyzing, decisioning, and creating hypotheses.
We want AI to do the heavy lifting on repetitive, mundane, yet critically important tasks and steps.
Over-relying on LLMs makes marketers too dependent on technology, potentially leading to a lack of problem-solving skills and critical thinking.
In scenarios where technology fails or is unavailable, a team without robust analytical skills may struggle to perform effectively.
By understanding all the risks, marketers can better strategize how to use LLMs and also AI Agents, making sure they complement human creativity and insight rather than replace it.
A balanced approach is always better, and especially when you want to build a trustful connection with your audience–, assuming they are also humans.
5. Privacy and Security Concerns
Moreover, the use of AI should be guided by ethical principles that prioritize privacy, ensuring that the systems respect and uphold the confidentiality and integrity of proprietary data.
If we follow best practices, we can harness the benefits of AI while safeguarding the privacy of any individual or company information, and continue to foster a trusting relationship between the technology itself and users of it.
The Future of LLMs and AI Solutions for Marketing
In the words of Shiv Singh, who recently authored the book AI for Dummies, if you aren't running towards AI, you will soon be out of a job. While that sounds scary to many, there is also a "steady-as-she-goes" approach that everyone can start doing today.
Assuming you have already used an LLM for a specific task, you are now on the path to getting immediate results and hopefully getting work done and time back in your day. Or at a minimum, you're becoming more knowledgeable.
If we ask ChatGPT's advice on how to adopt AI, it gives us pretty good advice.
Then, we apply some real-world human experience to that.
Here’s the Future of AI Usage in Marketing Teams
Focus on the use-case. Choose the Right AI Tools for the Team.
Pick the right tool for the job. Also, be careful about over-reliance on the AI tool and always put your creative human mind in the middle.
Ask how the entire team can benefit and learn. No more going solo.
Learn together. Re-use and share across the full team on the optimal solutions for the task.
Let's assume every software solution you already use will have LLMs and AI capabilities built in.
Start using those and take the beginner, more proficient, and advanced route along that path. It takes time.
Join virtual classes and attend events or join communities of like-minded pros.
Are the results proving and delivering value?
Measure your output and success. The beautiful promise of get work done faster is very attractive, with high-quality, excellent work, of course.
Don't forget to measure what you actually accomplish. Report to all relevant stakeholders.
Adjust existing processes. They won't disappear overnight.
It's ridiculous to believe that you can wash away all the amazing effort you've already put into the existing systems, tools, and processes.
Perhaps you've customized SaaS solutions like Salesforce or spent months tailoring Hubspot.
Now you're investing in Clay, which takes many weeks to months to see returns.
Take a closer look at where you have gaps and where you can truly start to use AI to get time back. Washing away your SaaS "run the business" apps, housing critical data will not be an overnight thing.
You can, however, begin to apply AI to those data sets for certain jobs to be done.
KPI metrics too
While LLMs are good at text-based questions and answers, you should also start to use AI for data computing or for getting answers on your important KPIs and structured data sets.
Don't ignore the fact that you can measure results much faster now with chat capabilities against the right data with results in tables and charts to share across the whole team.
Safety and costs
Work with your compliance and privacy teams internally to set any necessary guardrails.
Don't ignore this step or push it out farther, as it will eventually catch up with you. Stay safe and keep your proprietary info and data safe.
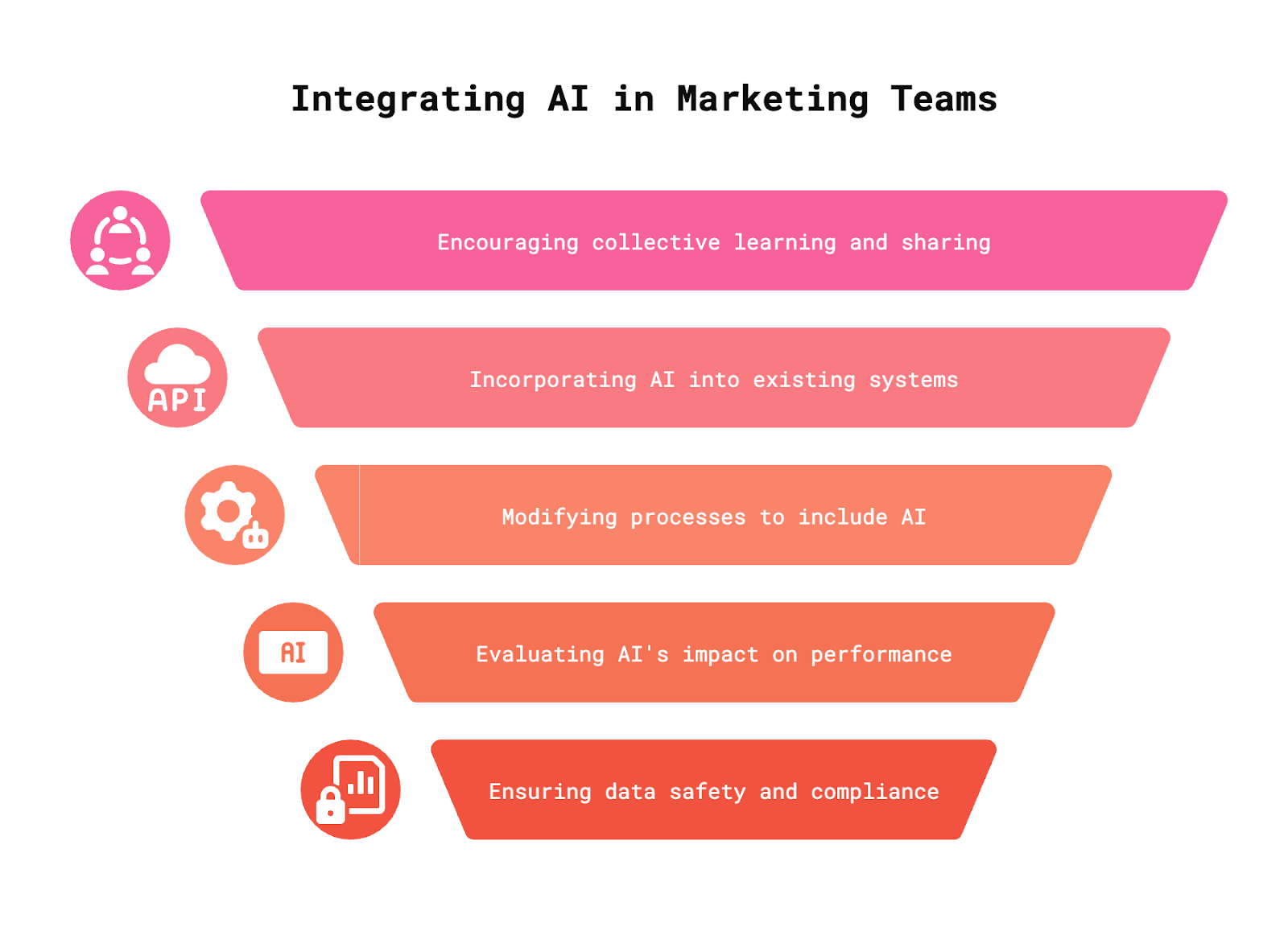
Want to Connect and Learn What MarkovML is up to?
Discover how MarkovML is building AI Agents to solve specific use cases and pains for growth and demand generation marketing teams that are under pressure every day to drive pipeline and accelerate revenue.
Contact me at: pankaj@markovml.com to find out more, and I'll walk you through a set of AI Apps and automated workflows.
Let’s Talk About What MarkovML
Can Do for Your Business
Boost your Data to AI journey with MarkovML today!