Revolutionize AI and ML: The Impact of Generative Adversarial Networks

As a knowledge worker in the domain of Artificial Intelligence and Machine Learning, understanding Generative Adversarial Networks (GANs) is pivotal to staying on top of advancements in this rapidly developing field.
This blog will give you an in-depth understanding of GAN and its potential effects on the AI industry.
Understanding GANs
A Generative Adversarial Network (GAN) is an AI model developed in 2014 by Ian Goodfellow and their team, designed for unsupervised machine learning to produce novel data based on input data.
GANs comprise two separate networks- the generator and the discriminator. The generator crafts new data points while the discriminator evaluates them for authenticity. These two different neural networks are pitted against each other, creating a game-like scenario.
1. The generator network attempts to create fake data that looks real.
2. The discriminator tries to identify which data is real and fake.
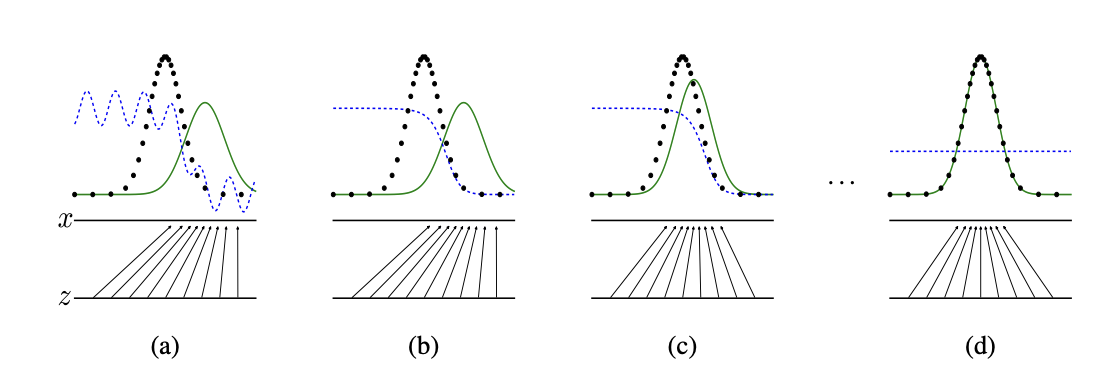
The result is that the discriminator network acquires the ability to classify these examples accurately. This knowledge is utilized to modify the generator network to produce more realistic data that can be distinguished from real data using the discriminator network.
The overall outcome of this process is a revolutionary enhancement in the realm of AI and Machine Learning, initiating a surge of advancements and applications.
Advantages of GANs
GANs stand at the forefront of machine learning innovations, introducing unprecedented advancements in AI creativity and data synthesis. These cutting-edge neural networks have opened a lot of possibilities, revolutionizing how we view and employ artificial intelligence.
1. Data Augmentation
GANs generate new data instances that resemble your training set, enhancing the accuracy and effectiveness of your AI-driven models. As knowledge workers, you can rely on GANs to furnish us with larger and more diverse datasets, thereby broadening our analytical reach.
Imagine you're a data scientist working on a healthcare project that requires a large amount of anonymized patient data. However, the dataset you possess is limited. Here, GANs can generate synthetic data that mimics the original yet maintains the key trends and patterns required for analysis.

Data augmentation can be applicable to audio, text, images, and any other types of data.
2. Image Generation and Realism

One of the astounding GAN applications is their ability to generate life-like images. They can create animations, devise virtual environments, and even assist architects in AI-driven simulations. The competitive nature of GANs helps them achieve a level of visual realism rarely seen in other AI technologies.
For instance, consider a game design company that has recently tapped into GANs' potential, using them to craft vivid 3D environments for their latest game release. The GANs can analyze countless photos of natural landscapes and generate stunningly realistic terrains.
3. Style Transfer and Image Translation
With GANs, the sector of art and design has witnessed a surge of AI creativity. From transforming photographs into paintings to converting zebra images to horse images while preserving background details, GAN's style transfer and image translation capabilities are nothing short of magical.

Consider this: you're an interior designer tasked with imagining a new space. With GANs, you can visualize various aesthetics in real-time, swapping a classic French set-up with a modern contemporary one in a snap while keeping the architectural backdrop intact.
4. Conditional Generation
Conditional generation is another GAN marvel. It allows the generation of targeted data types, providing more control over output generation. For instance, you can use this side of GANs to synthesize specific clothing styles in fashion design.
Imagine you're a fashion designer with an idea for a new clothing line but lack the resources to create prototypes. With conditional generation powered by GANs, you can specify parameters such as color, material, and style. The AI will then generate detailed, realistic images of the clothing line, giving you a virtual prototype.
5. Text-to-image Synthesis

The text-to-image synthesis capability of GANs is another game-changer, executing an area of AI that has always posed significant challenges. On providing a textual description, GANs can generate a corresponding image, opening up endless possibilities for creative professionals.
Imagine a scenario where a knowledge worker inputs a written description, such as "a yellow bird with black wings perched on a cherry blossom tree." An image corresponding to that description would be generated using the text-to-image synthesis capability of GANs.
Challenges and Ethical Considerations

The journey of GAN research directions takes us through various challenges and opportunities.
Whether it's creating AI-driven simulations or implementing configuration strategies, every side of this technology brings forth numerous aspects for exploration. Let's have a look at some of the major challenges:
1. Mode Collapse
Perhaps one of the most challenging aspects of GANs, mode collapse occurs when the generator starts producing similar outputs, severely hampering the benefits of data synthesis. Addressing this issue is vital to unleash the potential of GANs in producing diverse and novel solutions.
For instance, a GAN trained for creating unique facial images might end up generating nearly identical faces, thus showcasing the problem of mode collapse.
2. Training Instability
Machine learning innovations are not immune to instability in training - a common setback in GANs. The delicate balance between the generator and discriminator can result in unstable training, reducing the efficacy of GAN-based applications.
For instance, an imbalance in generator-discriminator dynamics might lead to corrupted image synthesis, hindering the performance of face recognition applications.
3. Evaluation Metrics
Evaluating the outcome of generative adversarial networks is often not straightforward. Creating an objective methodology for measuring the performance of GANs is crucial for their adoption and enhancement.
For example, discerning the authenticity of images generated by GANs poses a significant challenge due to the subjective nature of their quality assessment.
4. Robustness to Input Variations
A resilient GAN model must withstand changes in input and produce consistent results. Building robust models plays a key role in unleashing the full potential of AI creativity.
For instance, instabilities may arise when a GAN model generates incorrect results from minor changes in the input, stifling the advancement of AI creativity.
5. Biases in Training Data
The essence of responsible AI development lies in the ability to identify and minimize bias. Biased training data in GANs could lead to skewed or partial outcomes, undermining the artificial intelligence trends meant to level the playing field.
For instance, racial bias in facial recognition GANs could unintentionally lead to less accurate recognition of non-Caucasian faces, frustrating users and perpetuating inequality.
6. Ethical Considerations
As we dive further into the revelatory world of generative adversarial networks, we encounter numerous compelling benefits but also face a host of challenges worth probing. The development and application of these advanced AI constructs entail a range of potential ethical issues.
As you navigate the dynamic landscape of future Artificial Intelligence trends, you'll notice that GANs are fueling a revolution in AI and machine learning. However, this remarkable progress also sparks intriguing dialogues about balancing innovation with responsibility.
7. Bias in Generated Content
GANs, like all AI systems, are as impartial as the data they're trained on. Biased input data can lead to biased outputs, and the complex inner workings of GANs can often obscure this bias. It's a situation that calls for responsible AI development and diligent data synthesis.
For instance, a GAN trained on discriminatory housing data may unfairly reject loan applications from marginalized communities, amplifying existing biases.
8. Misuse of Generated Content
With the power to create remarkably realistic outputs, GANs could easily fall into the wrong hands. Carefully monitoring and controlling the applications can minimize potential misuse.
For instance, deepfakes could be generated by notorious individuals to propagate misinformation or malicious content, posing serious ethical and security issues.
9. Privacy Concerns
In an era of privacy in AI, ethical concerns abound. According to Security Magazine's research, data privacy concerns rank first among 65% of organizations' top generative AI concerns. This underscores the need for responsible AI development.
For instance, deploying AI in healthcare without ensuring data privacy can lead to unwanted disclosure of sensitive patient information, causing substantial ethical dilemmas and legal issues.
10. Adversarial Attacks
As the name suggests, GANs can be instrumentalized for adversarial attacks, altering inputs to deceive AI systems. Understanding these risks is a part of crucial GAN research direction.
For instance, fraudsters could manipulate financial transaction data to mislead AI-driven fraud detection systems, causing catastrophic losses for businesses.
11. Environmental Impact
Training these large neural networks requires substantial computational resources and energy. Therefore, the environmental impact cannot be overlooked.
The immense energy consumption of AI model training significantly contributes to the escalating global carbon footprint— an impending environmental crisis.
12. Lack of Control in Creative Applications
AI creativity brought by GANs presents its unique set of challenges. While unlocking new possibilities, it reminds us of the need for meticulous control in creative AI solutions.
For instance, unchecked automated image generation might inadvertently generate proprietary or inappropriate content, ending up with potential legal and ethical breaches.
These challenges point out the need for more robust frameworks for AI and ML innovations, encapsulating not just capability but also responsibility, marking the way towards the future of GANs.
13. Advancements in AI Creativity
AI creativity is on a revolutionary path, constantly redefining possibilities. A recent Forbes report found that 84% of marketing organizations are embarking on AI and machine learning innovations to enhance their creative processes. This underscores the shifting paradigm towards AI-driven solutions.
Generative Adversarial Networks (GANs) spirit lies in its two-network architecture. These two networks always try to outwit each other in terms of creativity and intelligence. One fabricates data, while the other tests it for authenticity.
An internal duel ensures that the results already have a high standard. They create and refine designs all at once, thanks to the constant internal input.
GANs indeed can ignite a spark in AI Creativity by producing excellent quality data. A recent study by TCS has emphasized that top-notch data quality is the cornerstone of making AI a runaway success. And GANs are the vehicle that propels us towards that goal.
14. Revolutionizing Product Imagery
If you need to generate realistic images for your new products on your eCommerce site, GANs is your answer. Let's say you run a fashion e-commerce website and need multiple pictures of your products with different models.
Here's where the magic happens.
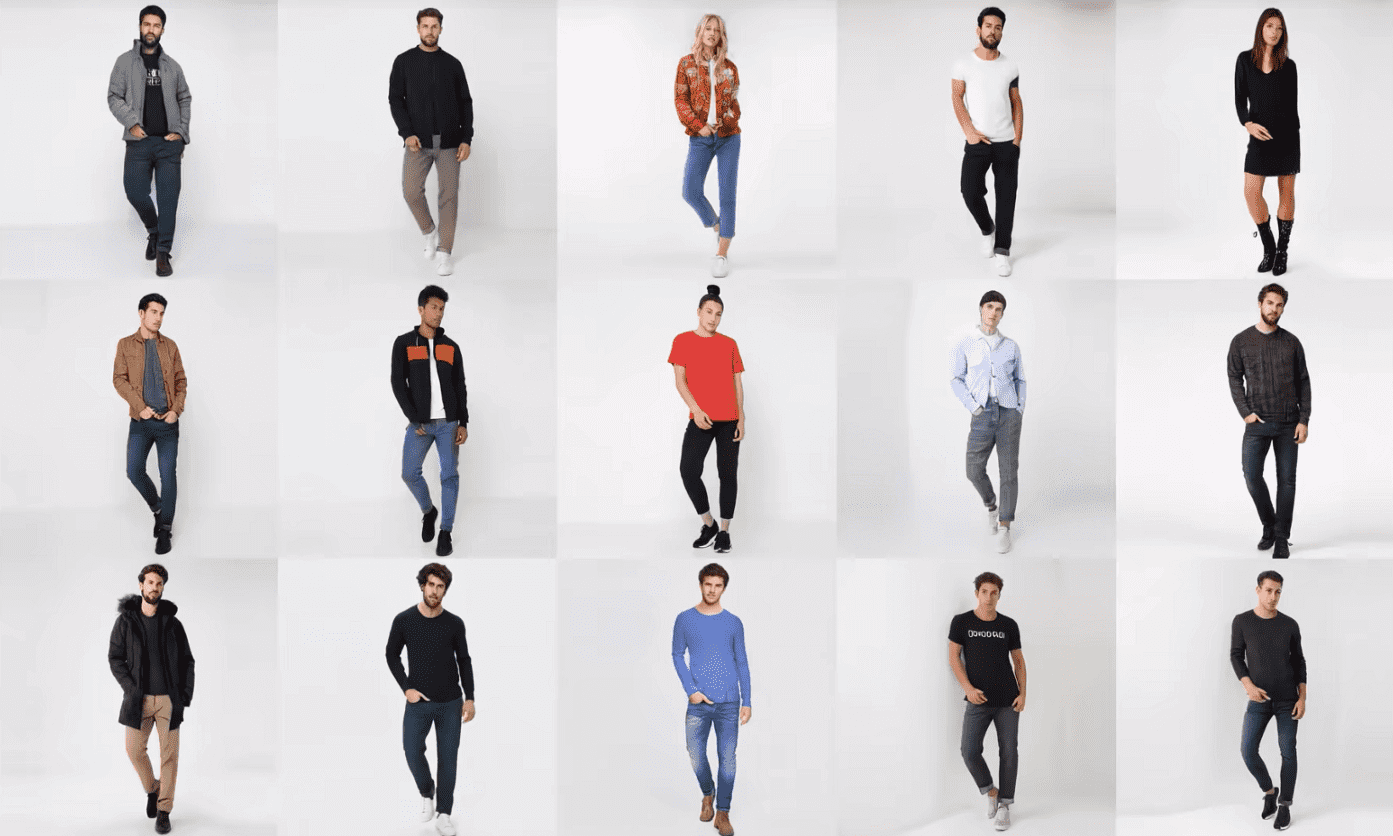
Using a fashion model as a guide, GANs can generate high-resolution photos and alter clothes and poses. They are able to replicate the same fashion model on a range of body shapes and attire. GANs are capable of producing models that both resemble and align with the target audience and brand.
With GANs and the use of a data quality measure, this seemingly daunting task becomes a breeze, yielding excellent results and supplying superior, labeled data quality.
15. Beyond Data Synthesis: GANs in Passive Entertainment
GANs, with their wide array of applications, are not just limited to data synthesis. They also significantly impact the field of passive entertainment. A prime example would be their use in AI-based simulations that help construct intricate virtual environments.

Imagine developing a sophisticated virtual city within a video game. The use of GANs could revolutionize this process, creating nuanced architectures, establishing diverse character models, and simulating dynamic weather conditions, thus ensuring an enriching and immersive user experience.
Impact on the AI/ML Industry
Delve deeper into the fascinating sphere of Generative Adversarial Networks (GANs). From fine-tuning data augmentation techniques and model training methodologies to redefining decision-making processes in businesses - GANs are truly changing the game.
1. Data Augmentation and Improved Training
Through GANs, data augmentation and subsequent training have significantly evolved, bringing major advancements to the AI/ML industry. With a creative approach to data synthesis, GANs can generate new data instances that amplify existing data, contributing to improved training of machine learning models and enhancing overall AI performance.
- GANs can generate a variety of synthetic data, which substantially boosts the training process in AI/Machine learning, rectifying the common issue of data scarcity.
- The enhanced training data improves the robustness and precision of AI models, resulting in sophisticated and reliable machine-learning innovations.
- GANs contribute to the diversification of training data, preventing overfitting and improving the model's ability to generalize from the learned data.
An example of such an application is in the field of healthcare, where GANs have been used for medical imaging. Faced with a shortage of relevant data, researchers used GANs to augment the available data, leading to better models capable of diagnosing conditions like cancer with more accuracy.
2. Advancements in Image Generation and Computer Vision
The impact of advancements in image generation and computer vision on the AI/ML industry is immense, driving significant technological innovations and opening new opportunities. These advancements have radically improved the capabilities of AI to understand and interpret visual data, thereby enabling more effective solutions.
3. Machine Learning Innovations
The use of sophisticated algorithms has enhanced the AI's ability to extract significant features from images and also to make sense of these features.
Consider this - a knowledge worker leverages machine learning to study patterns within complex radar imagery. The AI, powered by a sophisticated algorithm, does more than just recognize objects. It discerns patterns and behaviors undetectable to the human eye, resulting in a rich, high-quality dataset for stronger predictions.
4. AI Creativity
This has led to the generation of innovative applications in fields such as virtual reality, art, design, and advertising.
For instance, knowledge workers in the advertising industry are employing GANs to create dynamic and original content. They feed the AI random noise and marketing data, and the AI delivers unique advertisement layouts. This is revolutionizing creative process and output, providing flexibility and efficiency previously unimagined.
5. GAN Applications
The advancement in GANs, such as StyleGAN, has taken image generation to a whole new level. For instance, StyleGAN can create hyper-realistic faces of non-existent people.
These improvements have not only added to the sophistication of AI models but also expanded their applicability across industries.
6. Enhanced Creativity in AI Applications
As AI continues to evolve, AI creativity is a field making incredible leaps and bounds. Generative adversarial networks play a crucial role in these developments, enabling the creation of original, innovative content.
- GANs can produce imaginative designs and artwork like AI painting or designing logos. For instance, the "Portrait of Edmond de Belamy" was an AI-created piece of art sold at Christie's for a whopping US$432,500.
- The ability of GANs to generate realistic images can lead to advances in the animation and gaming industry. This leads to more immersive and life-like virtual experiences.
- With text-to-image synthesis capabilities, GANs can even convert narrative descriptions into vivid illustrations, finding applications in book publishing and content creation.
The power of GANs to create, imagine, and innovate is transforming what we perceive as possible in AI creativity.
7. Improved Business Solutions and Decision-making
Harnessing the power of generative adversarial networks can lead to innovative business solutions and aid in effective decision-making. They offer invaluable insights by creating diverse data possibilities and improving overall business processes.
- GANs facilitate the synthesis of new, realistic data, expanding the possibilities for training machine learning models. This, in turn, allows for better predictions and decision-making processes.
- Machine learning innovations brought forward by GANs, such as in anomaly detection, lead to more efficient business operations by identifying and mitigating potential risks in advance.
- They enable the creation of AI-driven simulations that can predict various scenarios, providing businesses with strategic insights for risk management and operational planning.
- GANs empower the development of responsible AI development, ensuring ethical usage of AI, which is crucial for businesses and their reputation.
For instance, a financial institution could implement GANs in its fraud detection systems. The GANs could simulate fraudulent activities to train the machine learning models, ensuring the system can accurately detect and possibly predict such activities.
Future Outlook
As we step further into the era of digital transformation, the future of generative adversarial networks (GANs) looks vibrant. GANs are postulated to drive the next wave of machine learning innovations.
Currently, various researchers are exploring new GAN research directions to overcome GAN challenges and enhance their capabilities. The Impact on AI and ML Industry is impressive, with the potential to transform sectors from healthcare to entertainment.
- By 2025, AI is expected to create 10% of data while GAN is being used that will alleviate privacy concerns - Gartner
- As per Gartner, GANs will generate 80% of all GenAI application interfaces by 2026.
Conclusion
As we explore the future of GANs, AI creativity, and ML innovations, the role of platforms like MarkovML becomes increasingly critical. From responsible AI development to data synthesis, our platform stands as a beacon guiding you through the misty unknown of the AI-driven simulations industry.
Whether you're seeking to unlock deep insights from your data or want to create intuitive, no-code generative AI apps, MarkovML is here for you. Our platform’s ability to automate intricate data workflows and foster collaboration among AI/ML teams adds immense value to your artificial intelligence trends journey.
Leverage the power of MarkovML to boost your speed of innovation and build better AI together. Start your journey towards an AI-empowered future today!
Let's usher in a new age of AI, with MarkovML leading the way.
Let’s Talk About What MarkovML
Can Do for Your Business
Boost your Data to AI journey with MarkovML today!