The Significance of Explainable AI in Machine Learning
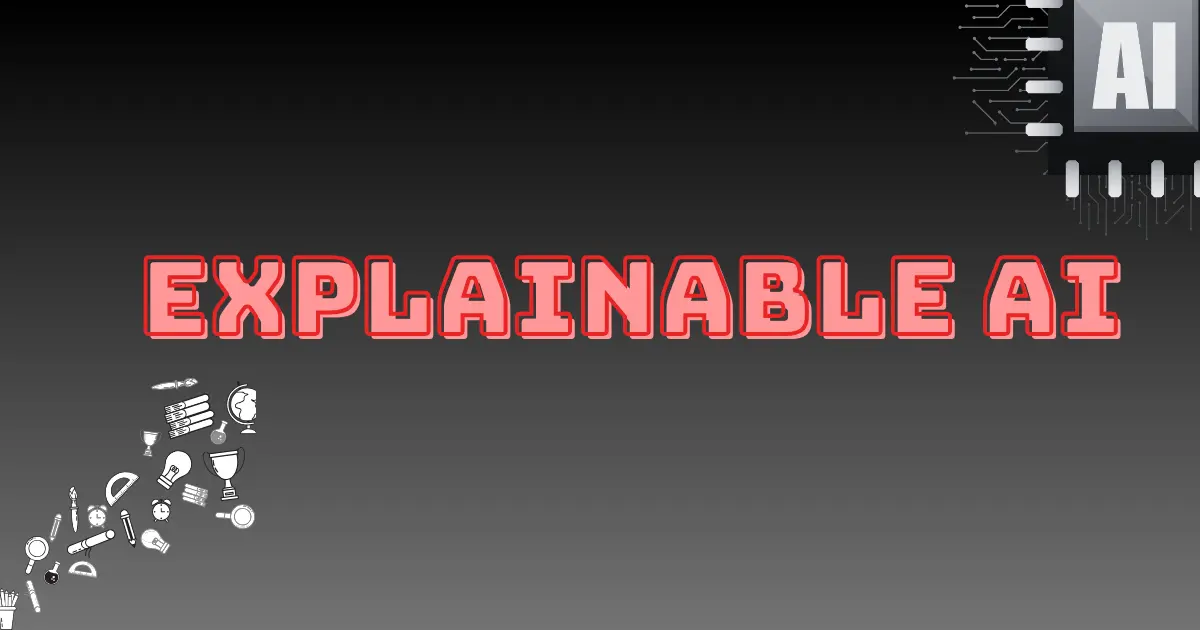
Can you trust AI systems to make meaningful decisions in your life or business? Do you understand how these AI systems will make these decisions? These questions have grown increasingly relevant as AI technology evolves, and the growth is projected to reach $1,811.8 billion by 2030, according to Forbes, leading to the development of Explainable AI (XAI).
This key advancement boosts trust and transparency in machine learning models, enhancing decision-making processes in domains such as healthcare and finance.
In this post, we'll discuss XAI, its techniques, challenges, and real-world applications and explore how AI can work for us in a transparent and trustworthy manner.
The Importance of Transparency in Machine Learning
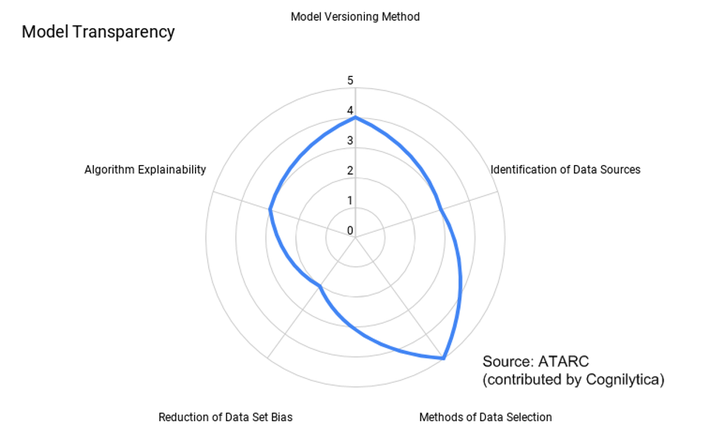
Transparency in machine learning, in combination with Explainable AI (XAI), is neither a fancy luxury nor an added feature — it’s a necessity for trustworthy systems. Still, the issue remains that while machine learning models excel at making precise predictions, they often act as a 'black box,' making it challenging for knowledge workers to understand and trust their decisions.
As machine learning becomes common in our everyday lives, we face the need to demystify these 'black boxes' using XAI Techniques. Transparency instills trust, and interpretable machine learning models help give users a clear understanding of the predictions they make.
Ethical AI implementation includes introducing XAI challenges in user-friendly AI interfaces. This makes AI systems more accountable and allows for fairer decision-making in sectors such as healthcare and finance.
In an era where AI impacts many decision-making processes, knowledge workers must understand how AI systems function. AI education for users is, therefore, essential. Real-world XAI applications have been crucial in improving transparency in sectors such as healthcare and finance.
MarkovML is at the forefront of introducing AI transparency solutions, promoting a human-centered AI approach. It assures the quality of labeled data for classification through its unique feature of data quality score. This empowers users to have a broader and clearer understanding of the workings of AI in various systems.
Trust in Machine Learning Models

Today, establishing trust in machine learning models is integral, and it's essential to comprehend the mechanisms that build this trust. Before we launch into tackling this expansive topic, let's kick off with a quick rundown of key aspects that help in mounting trust within the ML models.
1. Explainable AI (XAI)
This involves the application of techniques that increase the transparency of AI models, making them more understandable and relatable to their human users. For instance, MarkovML leverages Explainable AI to make its algorithms clear and accessible to its users. This goes a long way in cementing trust between the users and the AI models.
2. User-friendly AI interfaces
These play a crucial role in making complex AI and ML models easy to interact with, leading to increased acceptance and faith in these models.
3. AI Education for Users
Comprehensive education for knowledge workers about AI models, their working mechanics, potentials, and constraints is an essential step in ensuring trust in these models.
4. Human-centered AI
AI models designed and developed with a clear focus on the human user, taking into account aspects such as usability, accessibility, and ease of understanding, can help improve trust.
It's also critical to remember the challenges in this arena. XAI challenges still loom large - making the complex algorithms understandable to knowledge workers is not an easy feat. Then, there's the need for successful ethical AI implementation. Good ethics is essential in fostering trust, and it's necessary to ensure fairness, transparency, and inclusivity in AI models.
Moving ahead, healthcare and finance are two sectors where the need for trust is high and where the stakes on the line are massive. Emergent resolutions in these sectors have displayed immense benefits by successfully weaving an environment of trust between all stakeholders.
Ultimately, as we move towards an AI-driven future, the need to create a clear structure for trust-building is critical. It's a frontier that is not only beneficial but also mandatory for the successful, ethical, and sustainable progression of AI and ML in our societies.
Understanding Explainable AI (XAI)
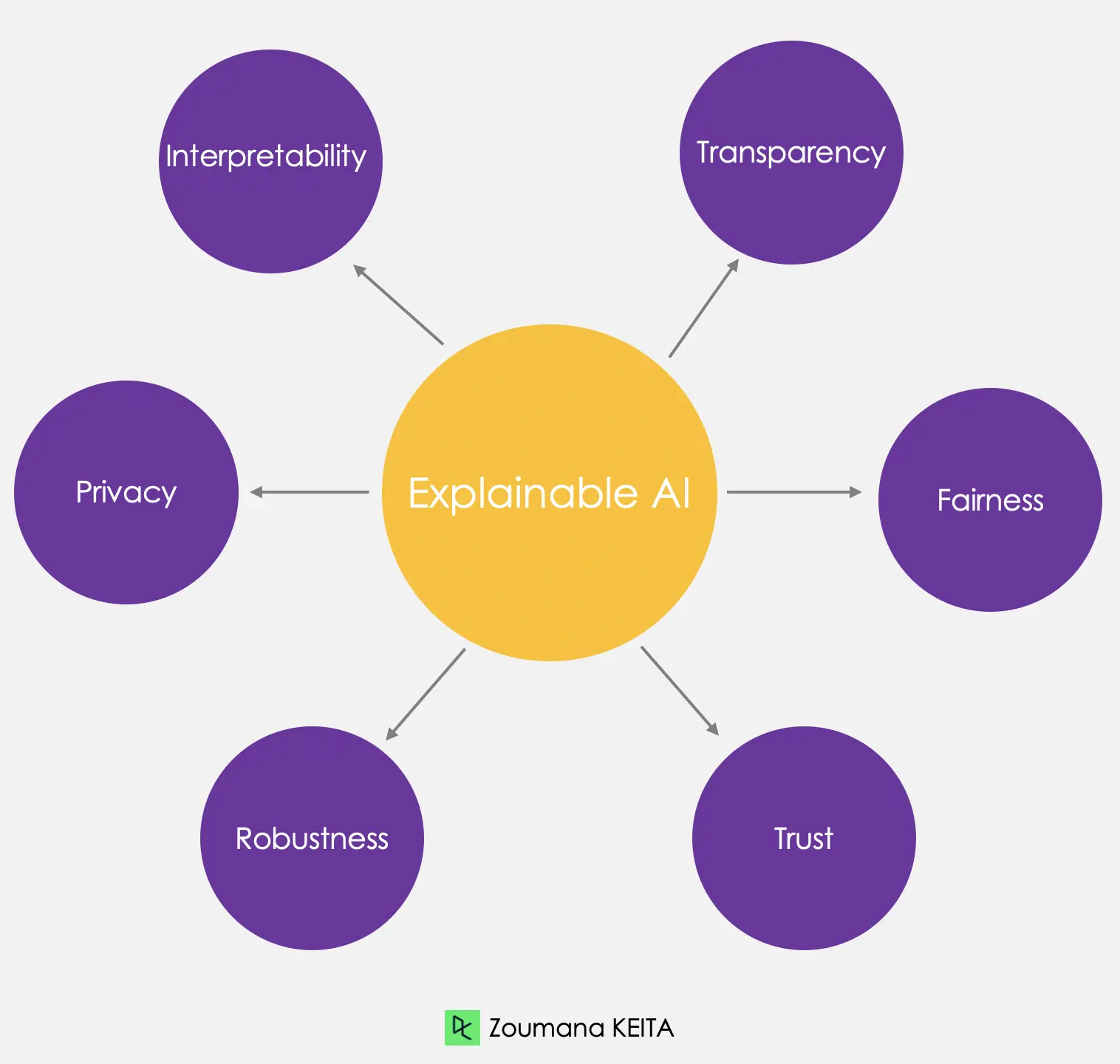
As a knowledge worker, you are part of a digital world where AI is prolific. However, how often do you find yourself questioning the decision-making process of AI? This is where Explainable AI (XAI) comes into the picture. XAI adds a layer of transparency to the often "black box" nature of AI models.
Explainable AI aims to create user-friendly AI interfaces where the algorithms behind the outcome become understandable and interpretable, not just to data experts but also to you - the end user.
It promotes ethical AI implementation by giving insight into how decisions are reached and addressing concerns of bias or unfairness. With XAI techniques, you can easily break down the process behind a machine learning model function, making these advanced technologies more approachable and trustworthy.
Challenges and Solutions
As we look further into the world of AI and its applications, it's important to address key issues surrounding the development and deployment of these emerging technologies.
While the potential of AI to revolutionize industries is indisputable, there's a dire need for clarity and accountability to ensure these systems are not just highly functional but also ethical and understandable.
1. Inherent Complexity of Machine Learning Models
Machine learning, a pivotal branch of AI, presents an inherent complexity that can be intimidating, especially to those with limited technical knowledge. Explainable AI (XAI) tackles this issue by developing models that are transparent and comprehensible, helping users navigate the world of AI without necessarily being experts.
2. Balancing Accuracy and Interpretability
The search for a balance between accuracy and interpretability is an ongoing challenge for AI practitioners. While highly accurate models are often less interpretable, simpler models that are easier to understand might not perform as well. Here, XAI techniques emerge as the solution.
3. Lack of Standardized Evaluation Metrics
Without standard metrics to evaluate AI models, it's hard to determine their fairness or efficiency. The call for ethical AI implementation underscores the need for such uniform measures that reflect the system's performance and ethical standing.
4. Interpretable Representation of Deep Learning Models
Interpretable machine learning is dedicated to creating deep learning models that users can understand and trust. These models not only promote AI transparency solutions but also foster a human-centered approach to AI technologies.
5. Addressing Bias and Fairness
AI has had its share of controversy around bias, pointing to an urgent need for fairer systems. Implementing user-friendly AI interfaces and educating users on AI through accessible, inclusive platforms such as MarkovML is an effective way to combat these issues.
6. User Understanding and Education
Critical to any technological advance is user understanding and education. By offering comprehensive AI education for users, it becomes easier to adopt AI technologies and use these tools effectively in various industries.
7. Scalability and Performance
An AI system's ability to scale and perform efficiently is vital. Emerging AI applications, such as AI in healthcare and finance, rely heavily on these attributes for successful implementations.
8. Integration with Existing Systems
For AI to usher in its full potential, integration with existing systems is key. Through real-world XAI applications, we can accomplish this seamlessly, making AI more usable and impactful in the process.
9. Regulatory Compliance
The regulatory landscape is catching up with AI's rapid growth. A commitment to AI transparency solutions ensures we are proactively shaping a future where AI systems are both highly innovative and ethically sound.
Applications of Explainable AI in Machine Learning
In recent years, there has been a significant surge in the adoption of AI technologies across various sectors. A key driving factor behind this is the progress made in machine learning models, notably the concept of Explainable AI.
The essence of Explainable AI is to create algorithms that generate decisions that not only have high accuracy but are also interpretable and trustworthy for knowledge workers.
This ethos is incredibly important in sectors such as customer service, finance, and healthcare, where decisions made by AI may have significant implications.
1. Customer Service and Chatbots
In customer service, Explainable AI has been significant in creating user-friendly AI interfaces for chatbots. The use of XAI techniques ensures that chatbots offer solutions that are easily understandable and trusted by customers, thereby advancing transparency and overall customer satisfaction.
2. Financial Decision-Making
The finance sector has greatly benefited from AI implementations, particularly in decision-making processes. Explainable AI bolsters transparency and trust, as the logic behind risk assessment and investment suggestions can be presented to the user, complying with regulatory requirements and ethical AI implementation.
3. Healthcare Diagnostics
In healthcare diagnostics, the need for AI transparency solutions is paramount. With life-changing decisions based on AI, XAI ensures not just accuracy but also the trust of patients, healthcare professionals, and regulators through clear, interpretable machine learning models.
The real-world XAI applications, particularly in medical imaging analysis, genetic research, and predicting disease patterns, are revolutionizing healthcare transparency
Future Trends and Development
Looking towards the future, Explainable AI (XAI) is poised to revolutionize the way we interact with technology. Driven by innovation in interpretable machine learning and the growing demand for ethical AI implementation, XAI will undoubtedly become an integral component of AI-powered systems. With XAI techniques at its core, it will provide AI transparency solutions tailored to a worldwide audience.
- In particular, user-friendly AI interfaces are to be a part of future development, ensuring seamless interaction and comprehension for knowledge workers alike.
- Moreover, the real-world XAI applications will expand beyond the healthcare and finance sectors, making AI more accessible.
- However, the pathway to Human-centered AI is not without obstacles, and XAI challenges are to be addressed head-on with users’ trust as a priority.
Conclusion
As the world embraces AI and machine learning more passionately, making this technology transparent, trustworthy, and user-friendly has become a pressing issue. In this quest, Explainable AI provides insights into machine learning models, offering a profound understanding of these intricate systems.
Embark on your journey of unlocking the true potential of AI with MarkovML. Dig deeper into data intelligence and management, unleash the power of generative AI apps, and streamline machine learning workflows with our data-centric AI platform. We foster AI/ML team collaboration to revolutionize decision-making processes.
Take the first steps to transform your data evaluation, boost the quality of labeled data for classification, and let your business reap the benefits of efficient AI implementation.
Let’s Talk About What MarkovML
Can Do for Your Business
Boost your Data to AI journey with MarkovML today!