Artificial intelligence has become an integral part of modern business operations. Besides streamlining processes and improving decision-making, artificial intelligence also improves the efficiency of businesses.
As artificial intelligence continues to develop rapidly, ethical concerns have taken center stage. The process of implementing ethical artificial intelligence in business poses several challenges.
With careful considerations and strategic planning, organizations can navigate these obstacles and build a foundation for responsible AI.
The Rise of Ethical AI in Business
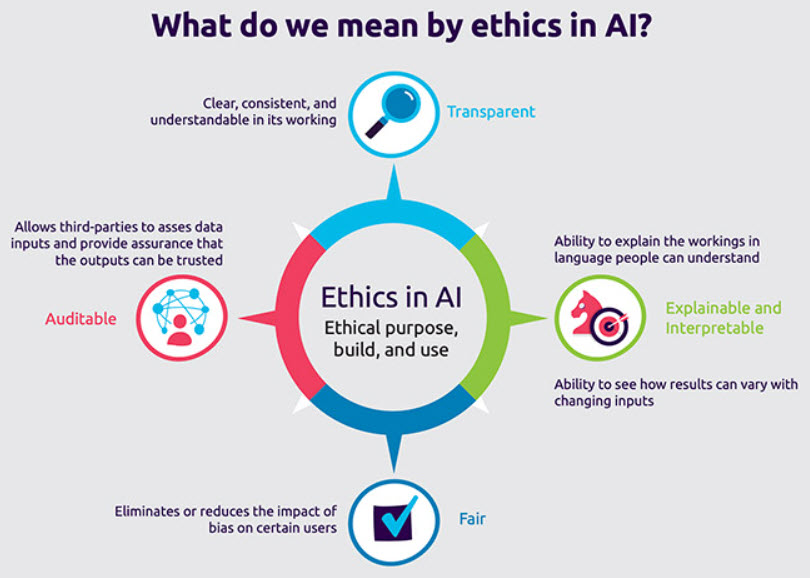
In recent years, there has been notable adoption of Ethical AI in businesses worldwide. Companies are increasingly recognizing the importance of incorporating ethical considerations into their AI systems to address concerns related to bias, transparency, and privacy.
For example, major tech companies like Google and Microsoft have committed to developing AI technologies that prioritize fairness and inclusivity. Ethical AI ensures that the algorithms are free from biases and that the decision-making processes are transparent and understandable.
Beyond moral imperative, Ethical AI development is a strategic move that businesses make to gain the trust of consumers for building long-term relationships. These organizations are incorporating ethics, promoting transparency, and integrating diverse data sets into the process of designing and developing AI systems.
Ethical AI is enabling organizations to create a more responsible and reliable future for artificial intelligence in the business landscape.
Challenges in Implementing Ethical AI
1. Algorithmic Bias and Fairness
The probability of bias is one of the main challenges in ethical AI implementation in global businesses. The fairness of AI systems depends on the data utilized to train them. If this data is biased, the AI systems also tend to be biased. The biases in data lead to discriminatory outcomes and negative results.
2. Transparency and Explainability
Lack of transparency poses a challenge as many algorithms are opaque and operate as black boxes. This makes it difficult for users to understand the decision-making processes. When AI systems lag in transparency and explainability, it hinders understanding, accountability, and trust among stakeholders.
3. Data Privacy Concerns
AI systems usually require a vast amount of personal data to train and improve their algorithm effectively. This enables these systems to recognize patterns, make accurate predictions, and perform complex tasks across different applications. Therefore, mishandling and unauthorized use of data that AI systems use to train their algorithms may lead to privacy breaches.
4. No-Code AI Platforms and Ethical Considerations
Users without extensive AI expertise may unintentionally introduce biases or overlook ethical considerations in algorithm design. It is important to balance accessibility with ethical guidelines to ensure responsible use while highlighting the need for ongoing education in the rapidly evolving arena of AI development.
Strategies for Ethical AI Implementation
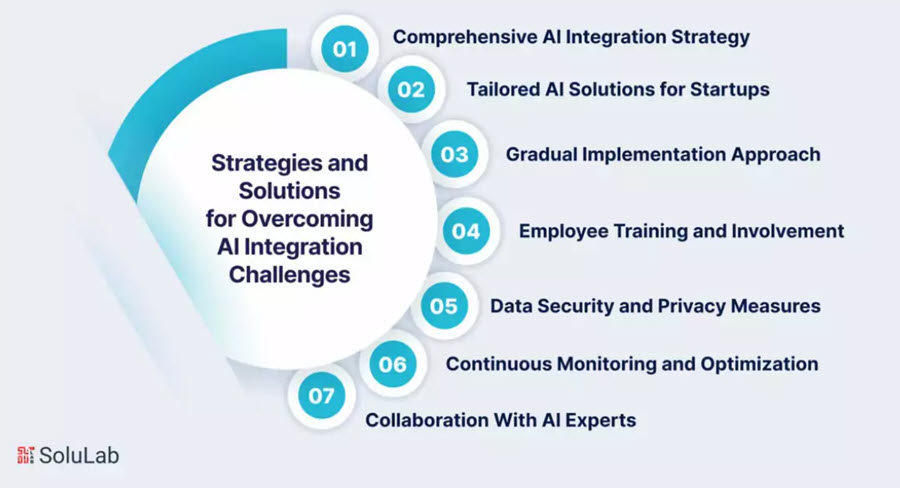
1. Diversity and Inclusion in AI Development
By incorporating diverse perspectives and inclusive datasets, organizations can effectively mitigate biases and promote fairness in creating AI Systems that cater to a broad range of users. For example, IBM’s Project Debater, developed by a diverse team, focuses on fair representation in its language models. It shows how inclusive practices can lead to unbiased AI systems.
2. Continuous Monitoring and Auditing
Regular assessment of AI systems helps identify and eliminate biases and ensure fairness. For example, companies like Accenture utilize ongoing audits to maintain ethical standards in their AI applications to foster transparency and accountability. MarkovML’s Connected Artifact GraphTM ensures that AI technologies align with evolving ethical norms to meet responsible standards.
3. User Education and Empowerment
AI education in businesses is crucial to eliminate barriers, no matter what AI tech stack you are working on. By educating users about AI capabilities, limitations, and ethical considerations, organizations can empower staff to make informed decisions. Google’s Teachable Machine project encourages users to understand AI concepts to enhance transparency and build trust.
4. Ethics-First Design in No-Code AI Platforms
Prioritizing ethical considerations in the development process to ensure unbiased results is referred to as ethics-first design. Platforms like Bubble.io focus on ethical design as they integrate features that prompt users to consider the ethical implications of their AI applications. It promotes a culture of responsible AI development and deployment.
The Role of No-Code AI Platforms in Ethical AI Adoption
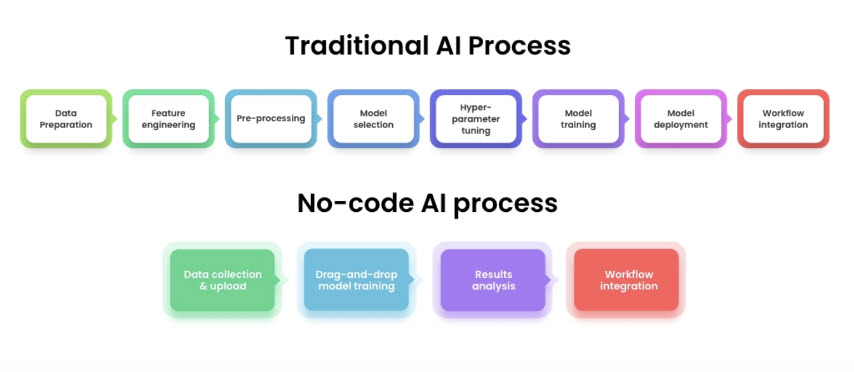
No-code AI platforms such as MarkovML play a key role in ensuring that AI is used ethically, even if you’re not an expert. These platforms are similar to user-friendly tools that allow people without deep technical knowledge to create AI applications without code. When it comes to business ethics, these platforms focus on doing things right from scratch.
Ethics-first design in no-code AI means that users think about what’s fair right before making an AI system. For example, suppose you want to use AI for hiring. An ethics-first approach in a no-code platform would remind you to consider if your AI might show favoritism and guide you to make it fair for everyone.
These platforms incorporate tools that help users think about important ethical questions, such as avoiding biases and being clear about how the AI works. It’s like having a friendly guide that reminds you to think about the feelings and needs of the team involved, ensuring your AI creation benefits everyone equally.
So, no-code AI platforms not only simplify things but also help ensure that AI is used responsibly and fairly for everyone.
Case Studies: Ethical AI Successes and Failures in Business
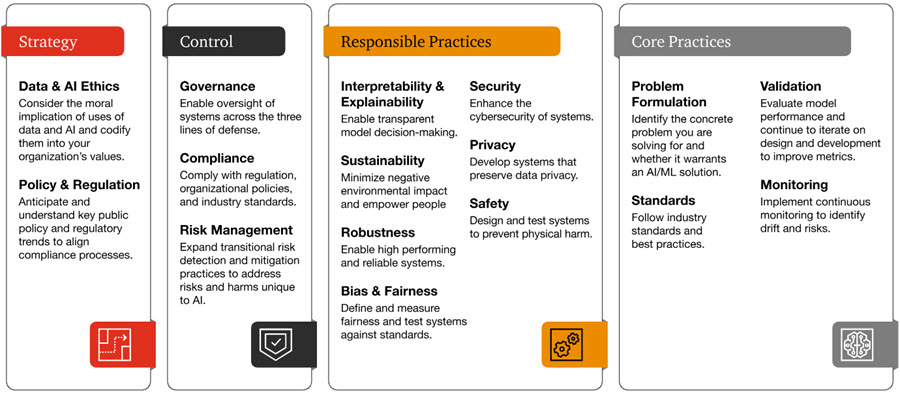
In this section, we will move on to the case studies that display the success and failures of Ethical AI implementations. Let’s first explore instances where organizations have excelled in implementing Ethical AI practices.
Ethical AI Successes in Businesses
Some of the examples of successful implementation of Ethical AI in businesses are as follows:
1. IBM’s Project Debater
This initiative by IBM demonstrates ethical AI success by promoting inclusivity in language models. The project aims to engage in unbiased debates on various topics. It displays the potential of AI to foster constructive conversations without favoring specific viewpoints. This approach contributes to a more open and diverse exchange of ideas.
2. Salesforce’s AI Ethics Framework
Salesforce has implemented an AI Ethics Framework, ensuring the responsible and ethical use of AI in its products. By incorporating principles such as fairness, transparency, and accountability, Salesforce sets a benchmark for ethical AI adoption. This explains the company’s commitment to building trust with users while avoiding potential biases in AI-driven functionalities.
Ethical AI Failures in Businesses
Let’s now discuss a few examples of ethical AI implementations that prove to be a failure for businesses.
1. Amazon’s Recruiting Tool
The tool faced criticism for gender bias and favoring male candidates. The failure of the project highlighted the risk of biased algorithms when trained on historically skewed data. The failure of the project emphasizes the importance of proper data cleaning to prevent discriminatory outcomes in the hiring process.
2. Google Photos Misidentification
Google Photos faced an ethical AI failure when its image recognition misidentified people of color as gorillas. This incident underscored the need for diverse and representative datasets to avoid perpetuating racial biases, showcasing the consequences of inadequate training data on AI system accuracy.
Conclusion
To sum up, the journey towards implementing ethical AI in business involves navigating significant challenges such as biases, lack of transparency and explainability, and privacy concerns. By adopting proactive strategies such as diverse data sets, ethics-first designs, and continuous monitoring, organizations can mitigate these challenges.
AI transparency, user education, and commitment to fairness are crucial for building user trust. The evolving AI landscape needs a paradigm shift, emphasizing ethics-first design and responsible practices.
By embracing these strategies for implementing ethical AI, businesses can address current challenges while laying grounds for future AI integration that aligns seamlessly with ethical principles. This enables organizations to foster trust, accountability, and equitable outcomes with MarkovML’s Responsible AI leading the way. Sign Up today and learn how you can scale AI without code.