The AI Workflow Revolution: The Top AI ML Tools to Know About in 2024
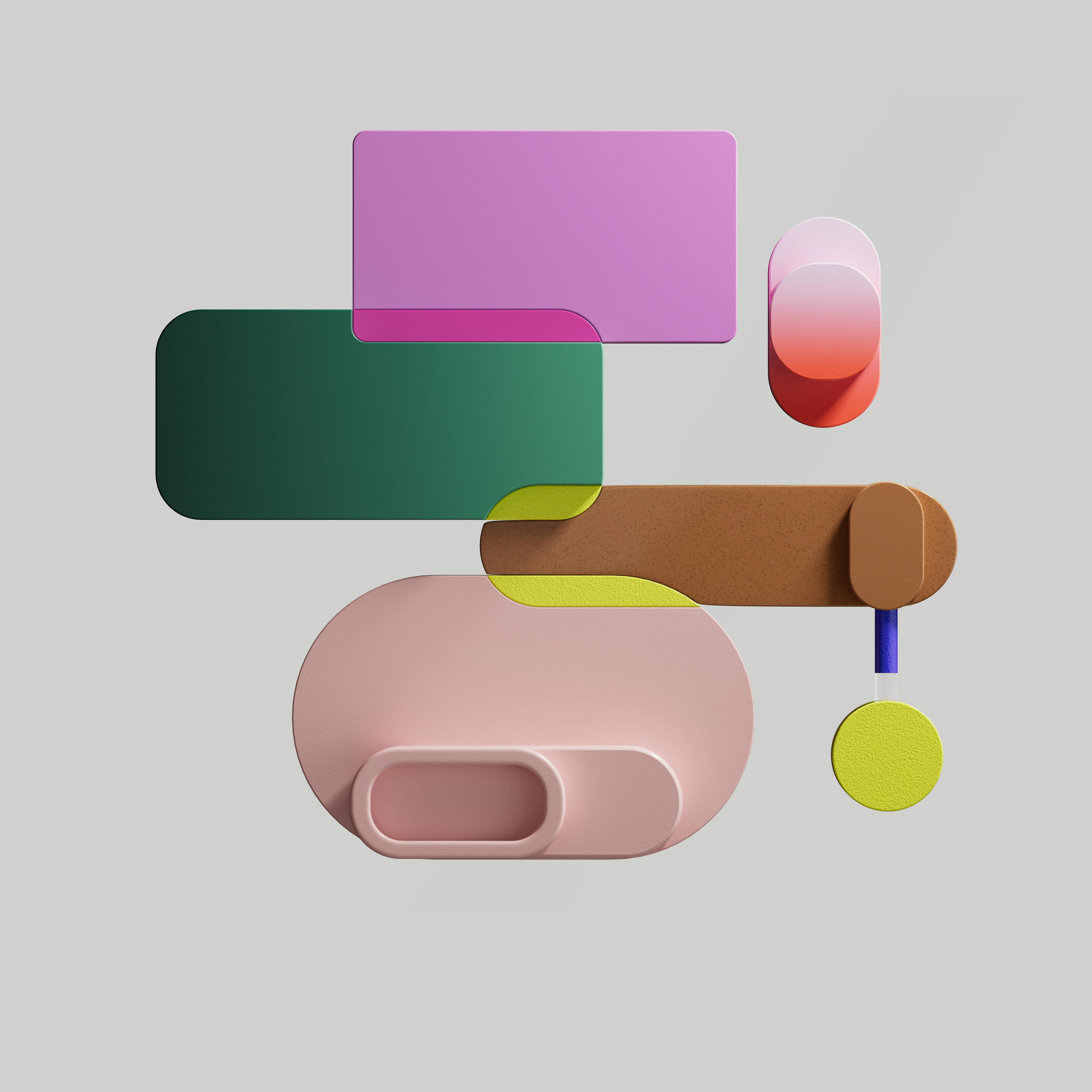
As we swiftly pace through the new millennium, we bear witness to the profound transformation technologies like Artificial Intelligence (AI) and Machine Learning (ML) have brought upon global industries. According to a 2020 report by the World Economic Forum, approximately 97 million people will be employed in the AI workspace by 2025.
As these cutting-edge technologies are continuously iterated upon and improved, the tools made as a result of their development have become essential for a data-driven workforce.
In this article, we will explore the riveting evolution of AI and ML tools and unravel their impact. By uncovering the top tools that stand at the forefront, we can catch a glimpse of the future trends that promise to shape the landscape of AI and ML.
The Evolution of AI and ML Tools
The evolution of AI and ML tools has written a narrative of relentless innovations and refinements that have compounded over time. The AI and ML model landscape has undergone a revolutionary transformation with the advent of cutting-edge hardware and sophisticated algorithms. According to a market analysis report by Grand View Research, the AI market was valued at $136.55 billion in 2022.
This advancement in ML algorithms has enabled the development of a variety of tools for meaningful uses, from insightful data analysis to the complexities of deep learning models. The range of use cases reflects an expansion in capabilities and broadening accessibility, enabling a larger audience to utilize the potential of AI and ML.
The impact of open-source collaboration is visible in the diversity of tools available. Collaborative efforts foster an environment where knowledge is shared and pushes the field forward. Libraries that facilitate machine learning tasks and sophisticated frameworks cater to a wide range of user requirements.
As the landscape matures, the trajectory of AI and ML tool development continues to be shaped by technological advancements and the need for resolutions to practical challenges. According to a market analysis report by Grand View Research, the global AI market is expected to reach $1,811.75 billion in 2030.
The Impact of AI and ML Tools
The impact of AI and ML tools can be witnessed across a myriad of industries, characterized by enhanced efficiency and informed decision-making. These tools extend far and wide in critical sectors such as healthcare, finance, and beyond.
- Healthcare: AI and ML tools have become valuable assets in the field of healthcare, helping optimize patient care and diagnostic accuracy. These tools have revolutionized how medical professionals can approach diagnosis and treatment planning with a degree of accuracy that is essential in a heavily regulated environment. According to a 2021 study by the University of Hawai'i, employing deep learning AI technology can improve breast cancer risk prediction.
- Finance: Automation in financial processes has become the norm, creating a more efficient and secure financial ecosystem. These tools make use of large datasets and complex algorithms to help institutions make data-driven decisions, contributing to the stability of financial systems. According to a 2023 Statista survey, one-third of the financial services surveyed have fully incorporated AI technologies into their businesses.
Beyond these sectors, AI and ML tools have become the foundation of efficiency, with routine tasks being delegated to algorithms and allowing human resources to be allocated to more involved objectives.
The Top AI and ML Tools
Let's now explore the top AI and ML tools you should know about in 2024, serving as beacons for enhanced efficiency and automation workflow implementation techniques.
1. TensorFlow
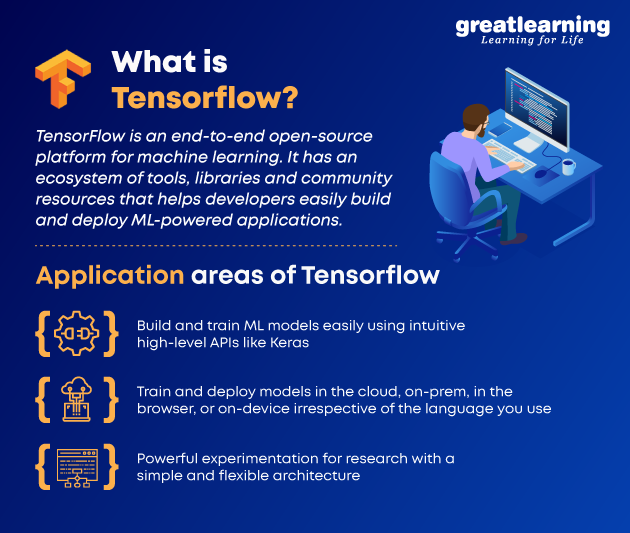
TensorFlow, developed by Google, is a free and open-source software library for machine learning and artificial intelligence. Known for its flexibility and scalability, TensorFlow supports a range of applications, from linear models to complex deep-learning neural networks.
TensorFlow.js for web-based applications, TensorFlow Lite for edge devices and mobile, and TensorFlow Extended (TFX) for end-to-end machine learning production are all part of its rich ecosystem.
2. MarkovML
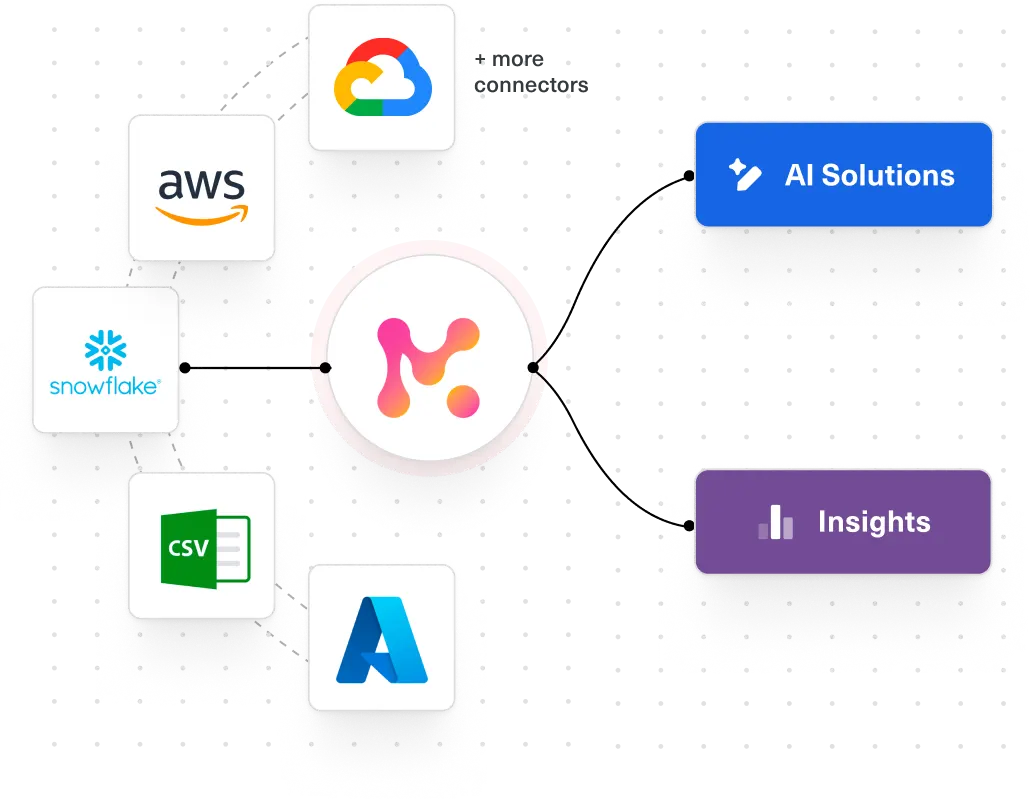
MarkovML is a cutting-edge platform that serves as a data-centric solution using the power of data intelligence and management, generative AI apps, and machine learning workflows.
MarkovML finds applications in natural language processing, speech recognition, and time-series analysis. The tool's ability to capture dependencies in dynamic systems and its predictive capabilities make it an invaluable asset. Moreover, it is a user-friendly, no-code AI platform for creating automated workflows and interactive model web applications.
3. PyTorch

PyTorch is a machine learning framework based on the Torch library, used for applications such as computer vision and natural language processing. It has gained popularity as a preferred choice for researchers and academics.
PyTorch's intuitive and dynamic interface allows for easy experimentation with neural networks. PyTorch supports dynamic computations, making it more flexible than static computation frameworks and enabling developers to modify models during training. It has a strong presence in the deep learning community.
4. Scikit-Learn

Scikit-Learn is a free machine-learning library developed to interact with Python numerical and scientific libraries, namely NumPy and SciPy. Its user-friendly interface and extensive documentation make it an excellent choice for beginners.
Scikit-Learn provides a wide range of algorithms for classification, regression, and clustering. Its simplicity and consistency during use help simplify the process of building machine learning models used in academics and industries for various real-world applications.
5. XGBoost

XGBoost is an open-source software library providing a gradient-boosting framework for a variety of programming languages, including C++, Python, and Java. It has become a powerful tool for machine learning tasks in structured data scenarios. By combining the outputs of multiple weak learners, it can provide robust and accurate predictions.
XGBoost is popular for its efficiency and speed, making it a go-to choice for data science competitions. Its popularity in the data science community is due to its ability to handle diverse data types, interpret feature importance, and easily integrate with other tools.
6. OpenCV

OpenCV is a cross-platform and open-source computer vision library that serves as an indispensable tool in the AI and ML landscape. OpenCV supports a vast range of image and video processing tasks and is designed to provide a common infrastructure for computer vision applications.
Its set of functions includes image recognition, object detection, and facial recognition. OpenCV's versatility and algorithms make it an essential tool for researchers, developers, and engineers involved with computer vision.
7. Hugging Face Transformers
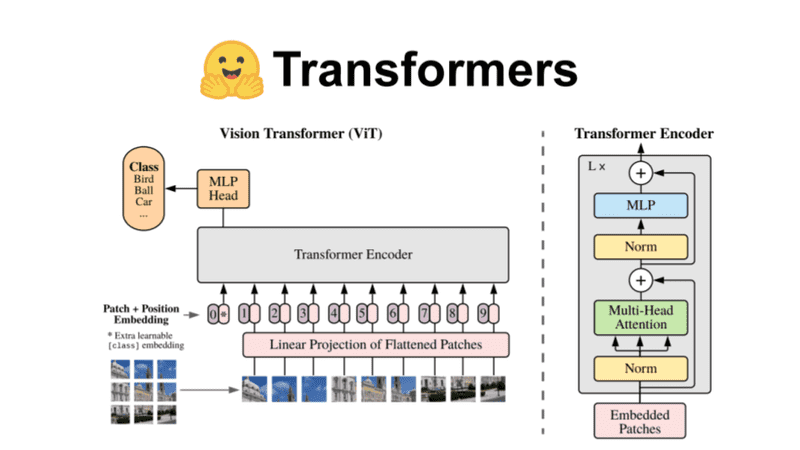
Hugging Face Transformers is a library that simplifies the use of pre-trained NLP models. With a collection of transformer-based models like BERT, GPT, and T5, Hugging Face Transformers empowers developers to utilize NLP capabilities in their applications.
The library facilitates easy deployment and refinement, enabling developers to build powerful language models with minimal effort. Its user-friendly interface and community support have led to widespread adoption.
8. DVC (Data Version Control)
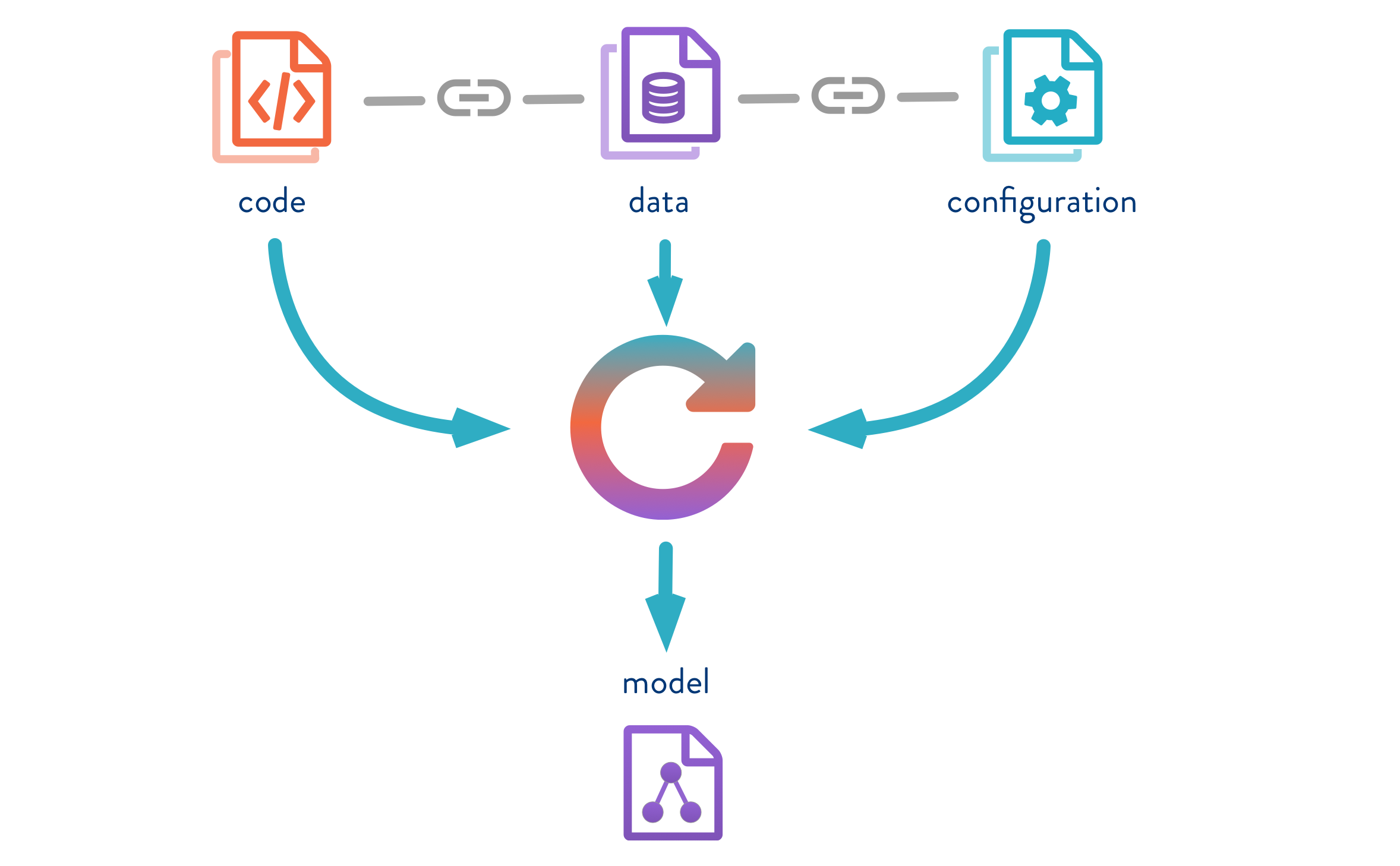
DVC is an open-source system for machine learning models and experiments that addresses the challenges that come with managing large datasets in machine learning projects. DVC allows data scientists to version control datasets efficiently, ensuring reproducibility and collaboration.
By decoupling data and code, DVC simplifies the tracking of changes in datasets, helping improve scalability and collaboration. Its integration with popular version control systems like Git makes it a great asset in machine learning projects.
9. Kubeflow
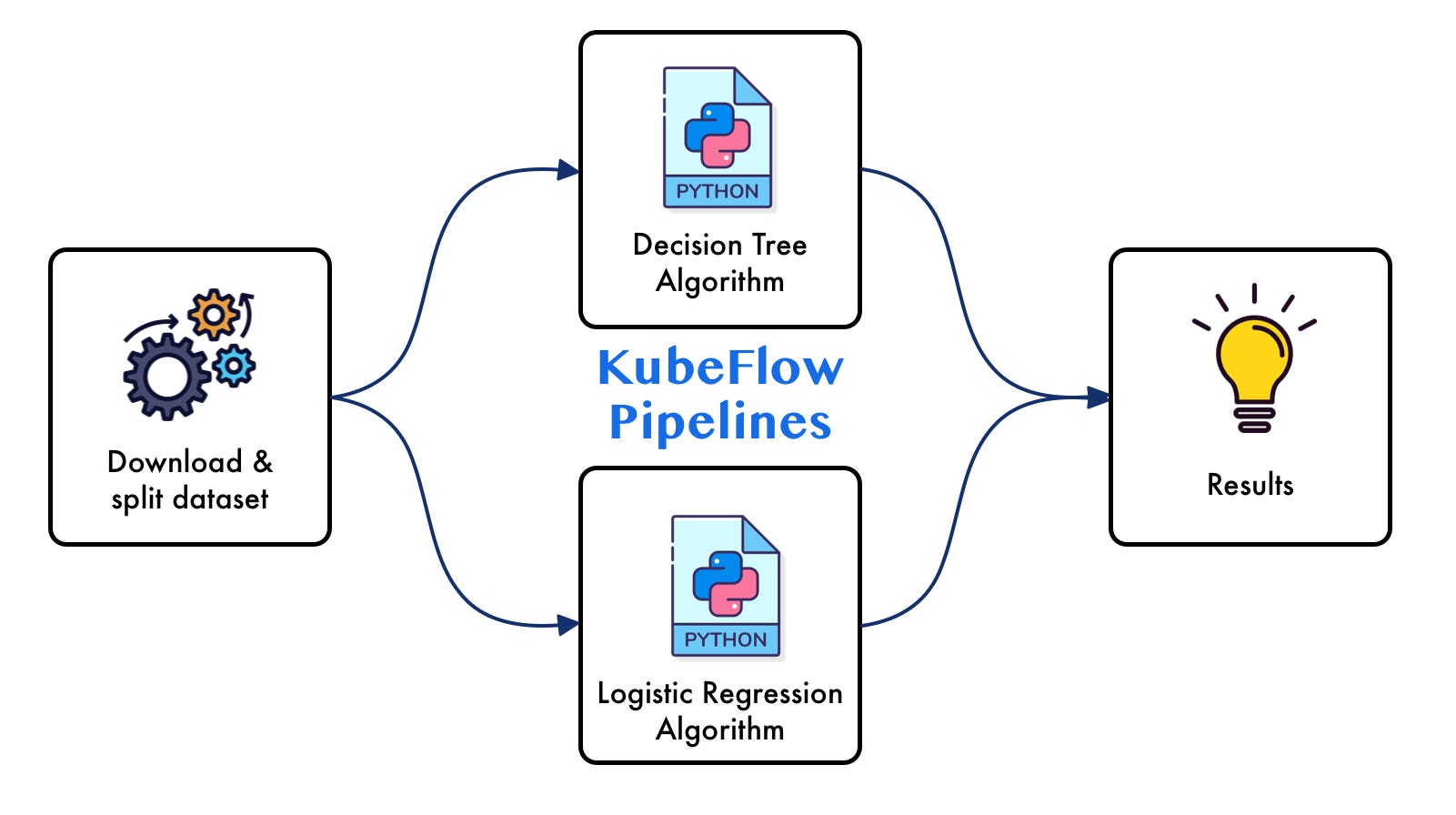
Kubeflow is an open-source platform designed to simplify the deployment and management of machine learning workflows on Kubernetes. With a consistent and scalable infrastructure, Kubeflow helps streamline machine learning models in production environments.
It includes tools for data preprocessing and model training, developing a seamless machine learning pipeline. Kubeflow's scalability makes it an ideal choice for organizations using Kubernetes for ML operations.
Future Trends in AI and ML
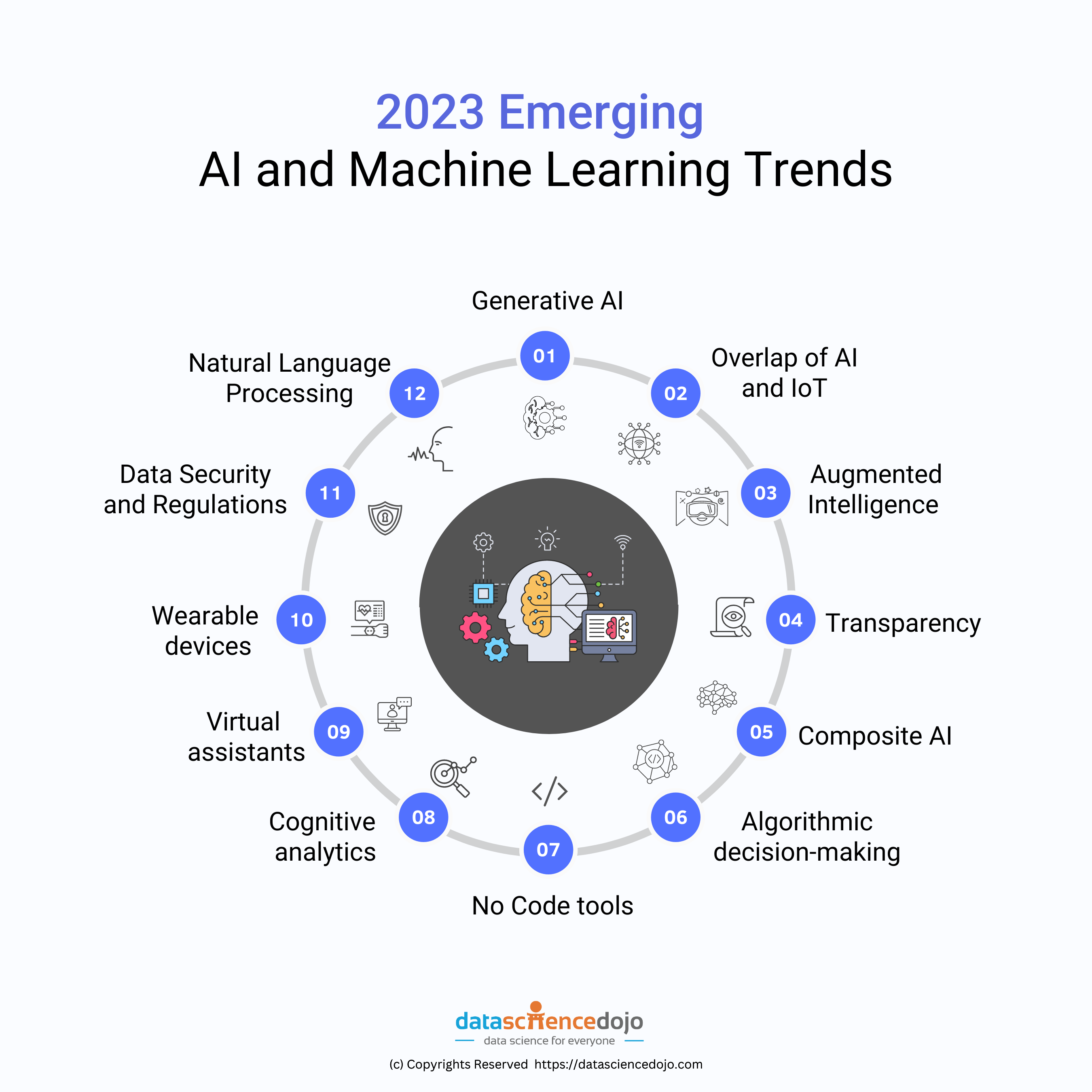
The future of AI and ML shows exciting possibilities facilitated by rapid and continuous advancements in technology. The integration of AI with edge computing and the increasing emphasis on responsible AI is expected to develop in the next generation of advancements.
AI democratization initiatives will play vital roles in making these technologies accessible and transparent. As they progress, we open up new opportunities for complex problem-solving. The convergence of AI and other emerging technologies will lead to innovative applications and further influence diverse sectors, such as healthcare and finance.
Innovative tools such as MarkovML serve as indispensable platforms for simplifying workflow automation and generative AI, which will help narrow the friction between professional exclusivity and broader access for knowledge workers.
Conclusion
The evolution of AI and ML tools has propelled the field further, transforming industries for a more efficient and data-driven future. The impact of these tools is evident in the efficiency gains, predictive capabilities, and transformative applications across domains. The top tools highlighted, from TensorFlow to MarkovML, showcase the diversity of AI and ML tools.
With continuous innovations, responsible development, and collaborative efforts, the future promises more remarkable achievements in the realm of artificial intelligence and machine learning algorithms.
When selecting the right tool, consider factors like the specifics of machine learning workflows and the complexity of datasets. For instance, MarkovML, an intelligent AI-powered platform, serves as a centralized hub, facilitating efficient AI and ML workflows and real-time collaboration.
As a dynamic tool featuring auto-data analyzers and generative AI app creation, MarkovML provides a solid foundation for knowledge workers to build a robust automated framework.
Let’s Talk About What MarkovML
Can Do for Your Business
Boost your Data to AI journey with MarkovML today!